Results
Solution
The business challenge
Developing proof of concept for the global deployment of predictive maintenance
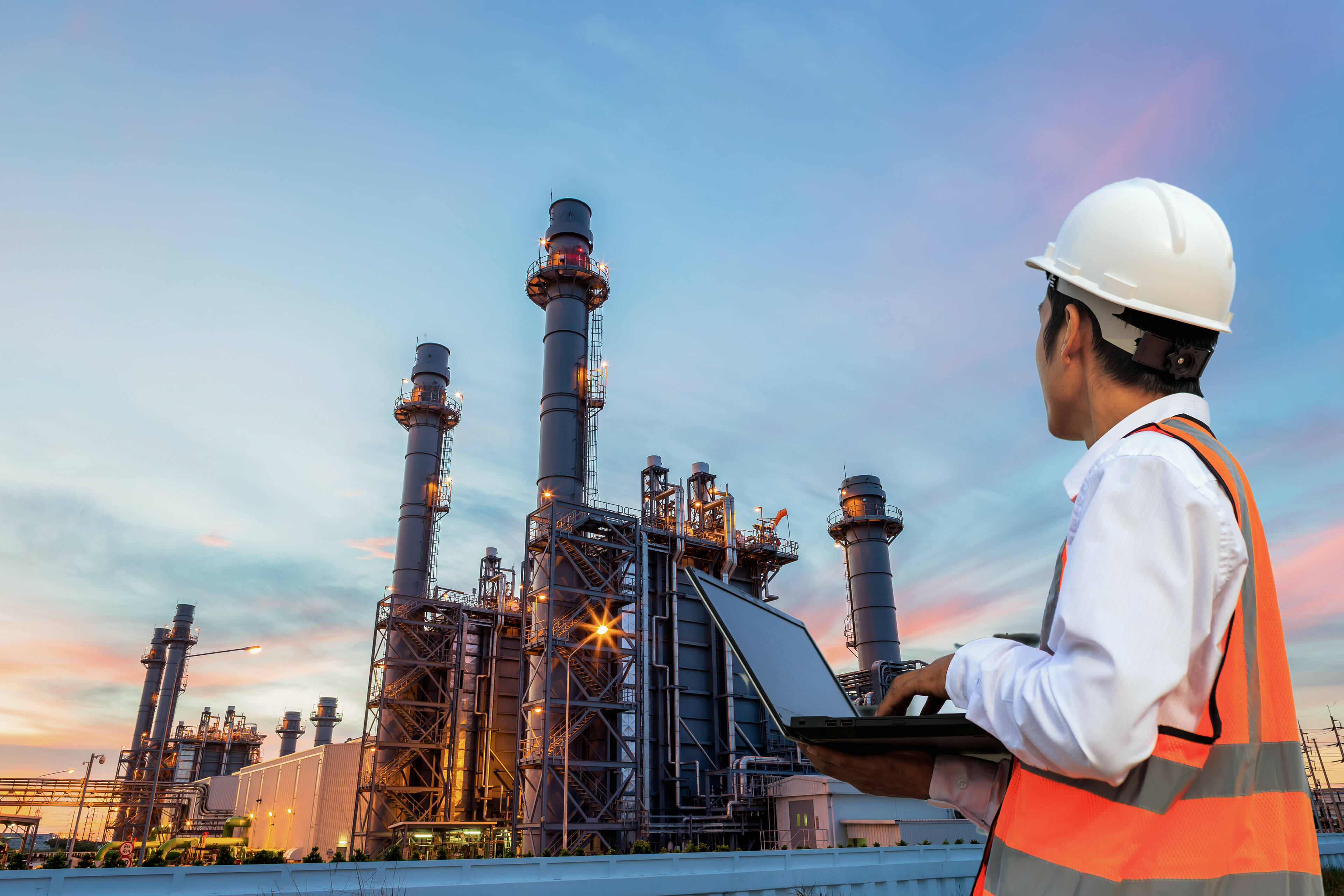
The business challenge
A multinational chemical supplier was experiencing a high number of disruptions at its production facilities due to unplanned repair and maintenance work.
This was creating a high percentage of reactive work orders – accounting for 43% of all its maintenance tasks – which was negatively impacting its efficiency. These work orders required 2-2.5x more operational time than planned jobs as materials needed to be prepared ad hoc, and necessary assets and permits weren’t readily available at the time of the interventions.
For this reason, the company wanted to reduce the amount of unplanned maintenance taking place by developing a digitally enabled predictive maintenance solution for its global operation. This solution would be able to anticipate problems with machinery before they became a major problem and allow maintenance work to be scheduled in advance – at times that would fit with the production schedule.
The solution
Before deploying a global solution, we advised the company to first develop and deploy a predictive maintenance solution at one site – with the intention of creating a proof of concept. Our team then worked in collaboration with the company’s maintenance, process, IT and data science teams to develop a system that would monitor the performance of 33 different pieces of machinery at its flagship plant in southern Europe.
We created a solution, built entirely from open-source technology, that was able to capture crucial machine data for analysis. Our team then trained algorithms to detect anomalies in these data streams, such as unusual machine vibrations or peaks in electricity consumption from motors, that would indicate wear and tear. A technical platform and customized data dashboard was also created to alert maintenance teams to potential problems that would require further inspection.
To ensure the project’s success we worked closely with the technical and engineering team to capture the insights and internal know-how. This helped us to select the correct assets for future roll outs of the solution and identified the locations where there would be a strong return on investment. With this knowledge, we presented the company with a business case for further deployment at various sites globally.
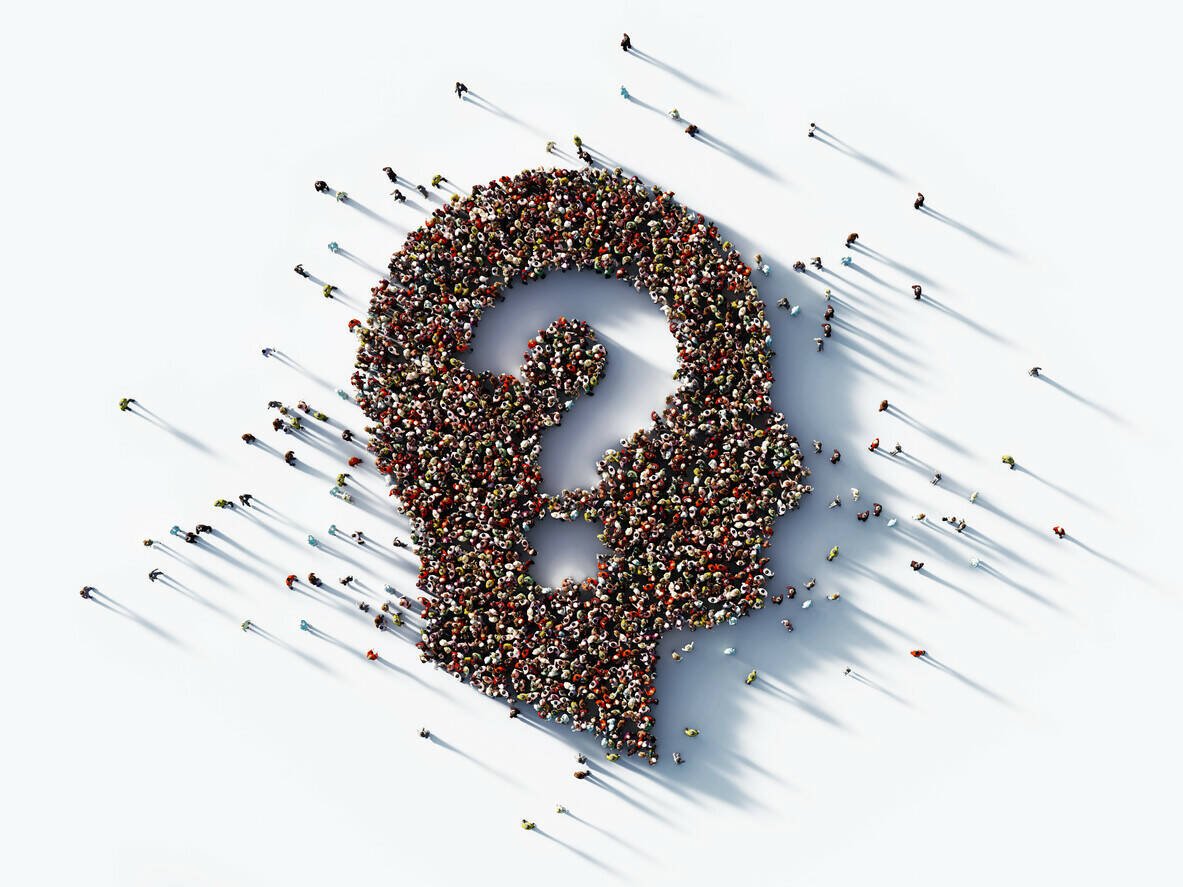
Results
The company achieved a predictive maintenance solution that managed to reduce maintenance costs at its flagship plant by 16%. This demonstrated the viability of its solution and proved the business case for internal roll out at eight further global sites.
Capturing know-how from within the organization ensured the relevant parameters were being considered and the solution was of practical beneficial to the end user. This engagement also ensured acceptance of the solution by the technical and engineering teams, who were then willing to integrate it within their daily working practices.
This system also successfully integrated sensor information with data coming from the company’s manufacturing execution system (MES) and enterprise resource planning (ERP) system. This enabled the creation of a solution that included a machine learning model – allowing the organization to predict machine performance over time.
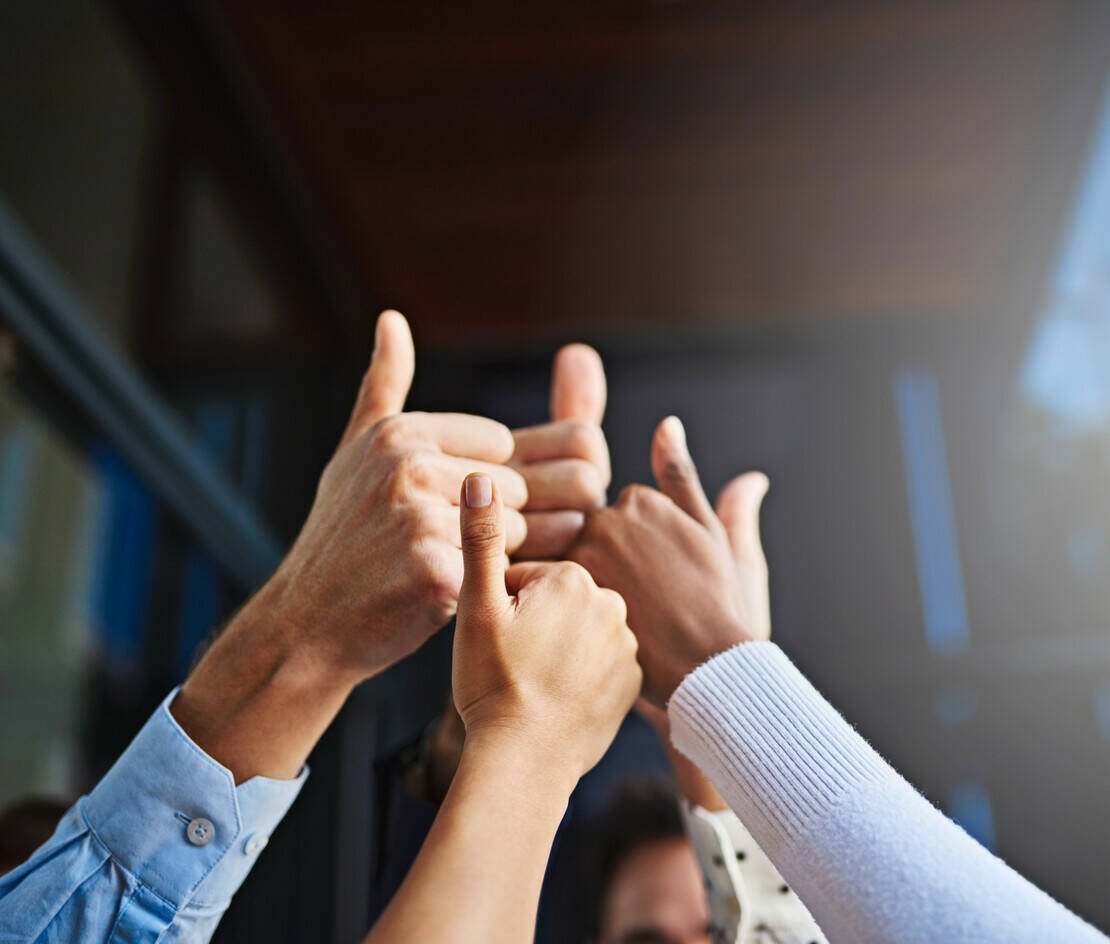
6 months
Project duration
Southern Europe
Location
Industrial Chemicals
Industry
The company achieved a predictive maintenance solution that managed to reduce maintenance costs at its flagship plant by 16%. This demonstrated the viability of its solution and proved the business case for internal roll out at eight further global sites.
Capturing know-how from within the organization ensured the relevant parameters were being considered and the solution was of practical beneficial to the end user. This engagement also ensured acceptance of the solution by the technical and engineering teams, who were then willing to integrate it within their daily working practices.
This system also successfully integrated sensor information with data coming from the company’s manufacturing execution system (MES) and enterprise resource planning (ERP) system. This enabled the creation of a solution that included a machine learning model – allowing the organization to predict machine performance over time.
Results
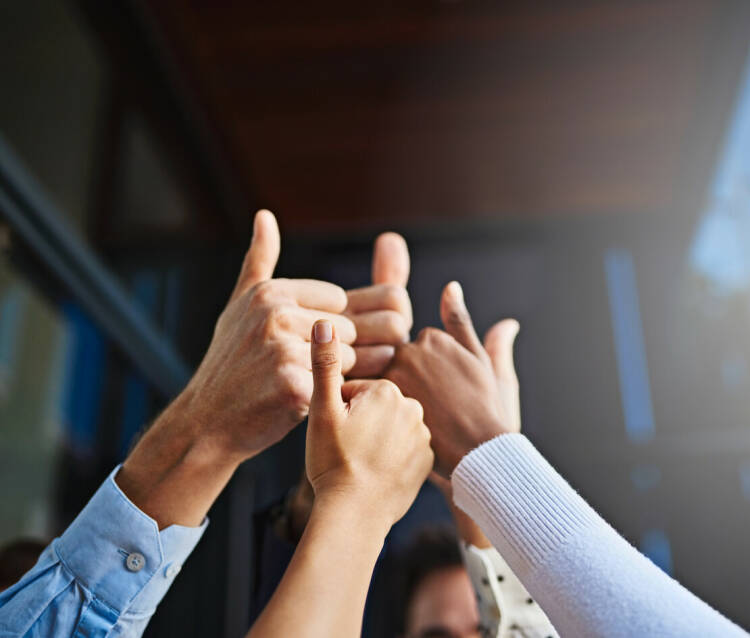
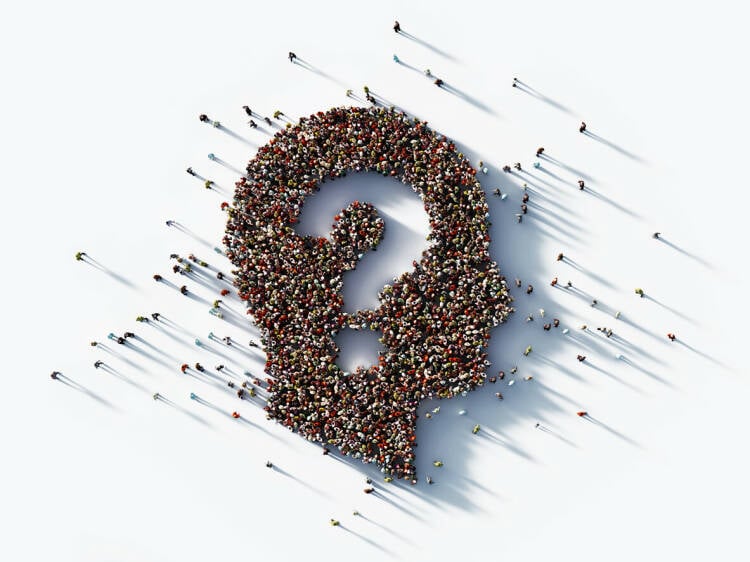
Before deploying a global solution, we advised the company to first develop and deploy a predictive maintenance solution at one site – with the intention of creating a proof of concept. Our team then worked in collaboration with the company’s maintenance, process, IT and data science teams to develop a system that would monitor the performance of 33 different pieces of machinery at its flagship plant in southern Europe.
We created a solution, built entirely from open-source technology, that was able to capture crucial machine data for analysis. Our team then trained algorithms to detect anomalies in these data streams, such as unusual machine vibrations or peaks in electricity consumption from motors, that would indicate wear and tear. A technical platform and customized data dashboard was also created to alert maintenance teams to potential problems that would require further inspection.
To ensure the project’s success we worked closely with the technical and engineering team to capture the insights and internal know-how. This helped us to select the correct assets for future roll outs of the solution and identified the locations where there would be a strong return on investment. With this knowledge, we presented the company with a business case for further deployment at various sites globally.
The solution
The business challenge
6 months
Project duration
Southern Europe
Location
Industrial Chemicals
Industry
A multinational chemical supplier was experiencing a high number of disruptions at its production facilities due to unplanned repair and maintenance work.
This was creating a high percentage of reactive work orders – accounting for 43% of all its maintenance tasks – which was negatively impacting its efficiency. These work orders required 2-2.5x more operational time than planned jobs as materials needed to be prepared ad hoc, and necessary assets and permits weren’t readily available at the time of the interventions.
For this reason, the company wanted to reduce the amount of unplanned maintenance taking place by developing a digitally enabled predictive maintenance solution for its global operation. This solution would be able to anticipate problems with machinery before they became a major problem and allow maintenance work to be scheduled in advance – at times that would fit with the production schedule.
Developing proof of concept for the global deployment of predictive maintenance
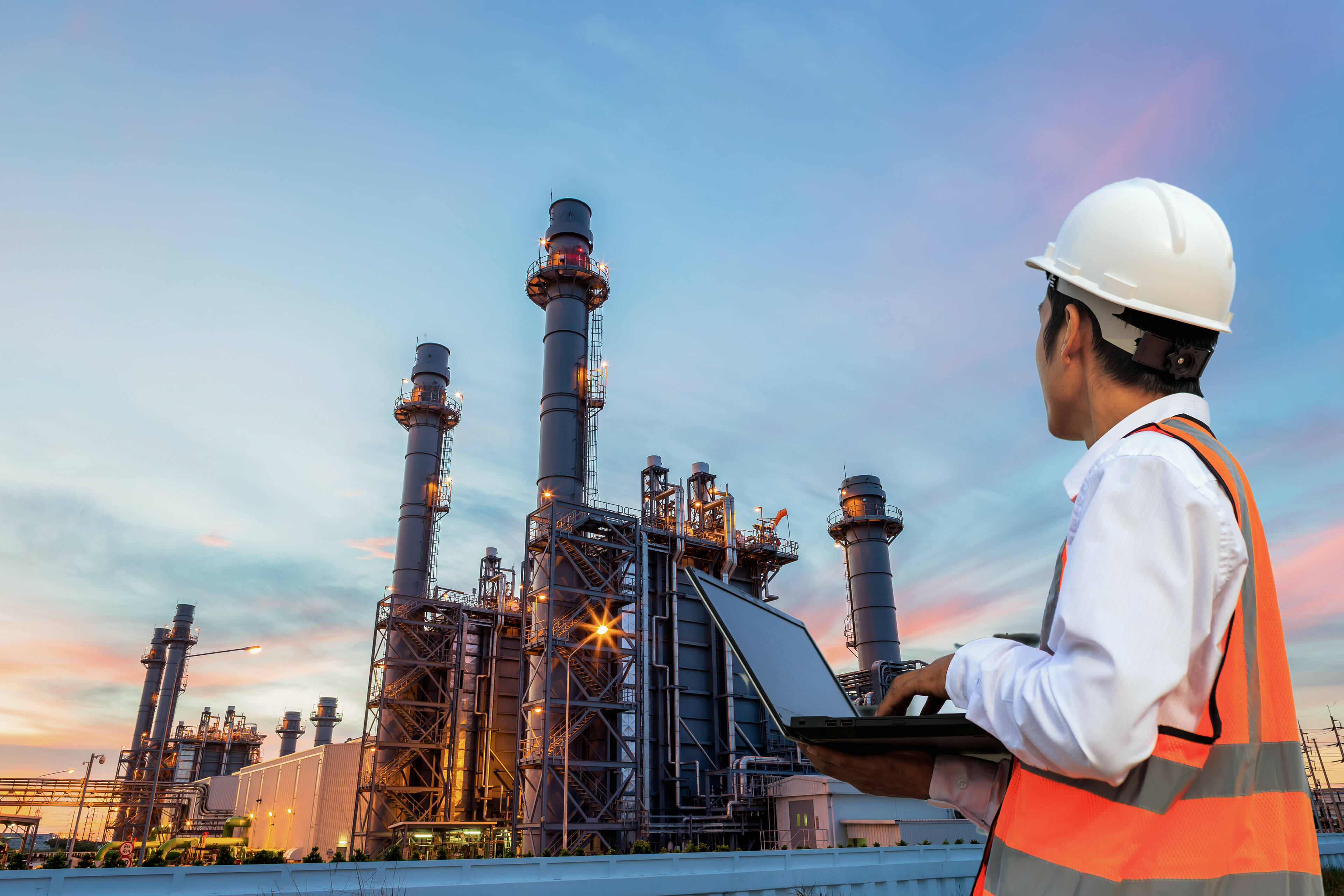